Our economy’s financial sector is using machine learning (ML) more often to support lending decisions that affect our daily lives. While technologies such as these pose new risks, they also have the potential to make lending fairer. Current regulation limits lenders’ use of ML and aims to reduce discrimination by preventing the use of variables correlated with protected class membership, such as race, age, or neighborhood, in any aspect of the lending decision. This research explores an alternative approach that would use an applicant’s neighborhood to consciously reduce fairness concerns between LMI and non-LMI applicants. Since this approach is costly to lenders and borrowers, we propose concurrent use with more advanced ML models that soften some of these costs by improving model predictions of default. The combination of embracing ML and setting explicit fairness goals may help address current disparities in credit access and ensure that the gains from innovations in ML are more widely shared. To successfully achieve these goals, a broad conversation should continue with stakeholders such as lenders, regulators, researchers, policymakers, technologists, and consumers.
Advancing Fairness in Lending Through Machine Learning
Advances in machine learning (ML) provide the opportunity to improve predictions that may expand credit access to more applicants. However, there is concern that gains from advanced models could accrue unequally between demographic groups or do little to reduce existing disparities in credit access. This research explores an approach using ML — paired with setting explicit fairness goals — that may help address current disparities in credit access and ensure that the gains from innovations in ML are more widely shared.
Illustrating the Fairness-Profit Tradeoff
Current regulation limits a lender's use of ML and aims to reduce discrimination by preventing the use of certain sensitive information in any aspect of a lending decision. This research contributes to the discussion around fairness in lending by exploring an alternative approach that uses ML and an applicant’s neighborhood to consciously reduce disparities in credit access among applicants living in (LMI) and non-LMI areas.
The focus of this research is fairness, defined as equal access to credit among applicants from LMI and non-LMI areas. We examine two approaches: improving models through ML and setting fairness goals.
- Improving models through ML makes predictions better in general but does not address disparities in credit access between LMI- and non-LMI-area applicants.
- Setting fairness goals achieves more equal access to credit between LMI- and non-LMI-area applicants, but it comes at a cost — more defaults among LMI borrowers harm those borrowers and reduce lender profitability.
The combination of using more advanced ML models and setting a fairness goal, however, can improve fairness and increase profitability because of improved default predictions using ML. This graphic illustrates the tradeoff between fairness in accessing credit and lender profitability.
Try using the dropdowns to change the model type and alter the fairness goal to explore how fairness and profitability change in this study. Each selection compares fairness and profitability outcomes with a simplified representation of the .
More details on Model Improvement, Fairness Goals, and Putting It All Together are provided below.
Model Type
Fairness Goal
Key Takeaways
Model Improvement
Why Does Model Improvement Matter? Are More Advanced Models Fairer?
One goal of improving a predictive model using ML is to better align its predictions with reality. Our results, like previous research, show that more advanced models improve predictions for all applicant groups. However, more advanced models do not significantly reduce existing disparities between groups. We find that creditworthy applicants in LMI areas have less access to credit compared with creditworthy applicants not living in LMI areas, even when more sophisticated models are used.
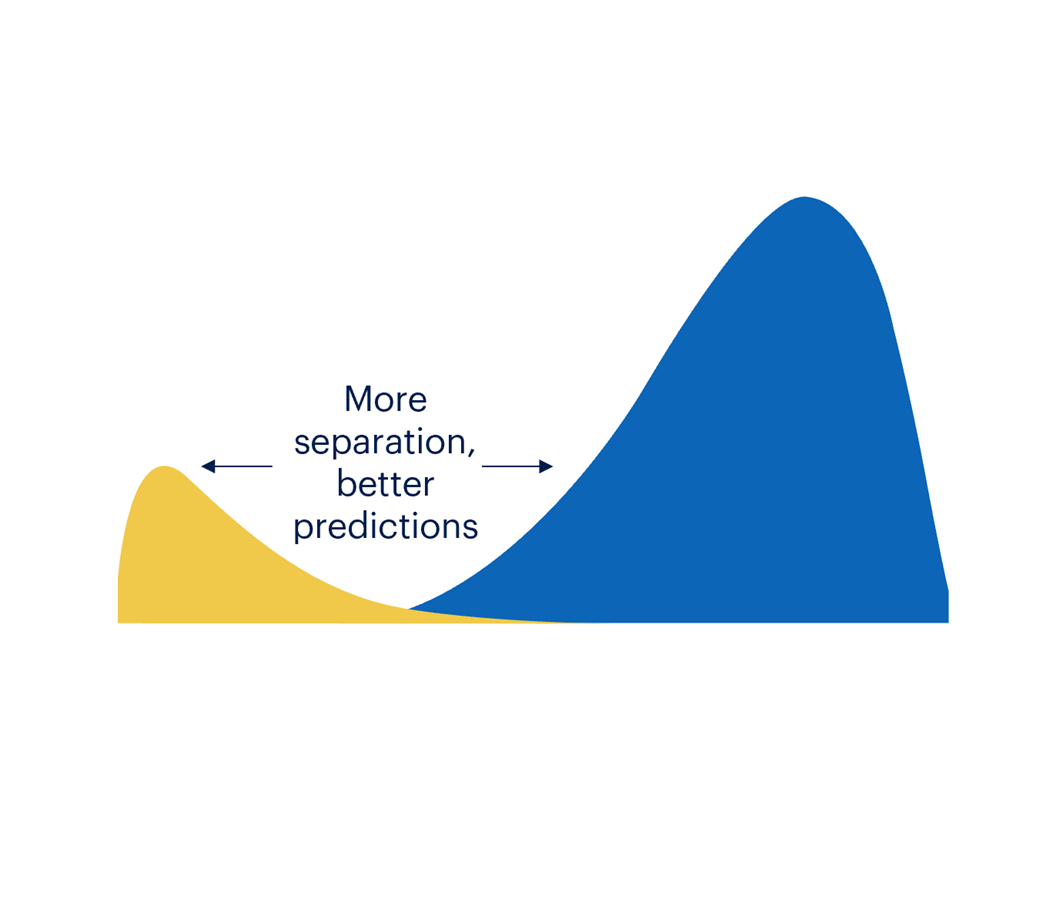
Fairness Goals
How Can Incorporating Group-Specific Thresholds Affect Fairness?
One solution to create more equal access to credit between groups is to have a different credit score requirement for specific groups of applicants (i.e., group-specific thresholds) that are set to achieve a fairness goal. For our study, this translates to lowering the credit score threshold in LMI areas rather than using a single credit score threshold for all applicants. This research considers how setting group-specific thresholds can achieve fairness goals while examining the effect on two important metrics: the (TPR) and the (FPR). Group-specific thresholds successfully achieve more equal access to credit among creditworthy applicants from LMI and non-LMI areas, but their use is associated with costs for lenders and borrowers.
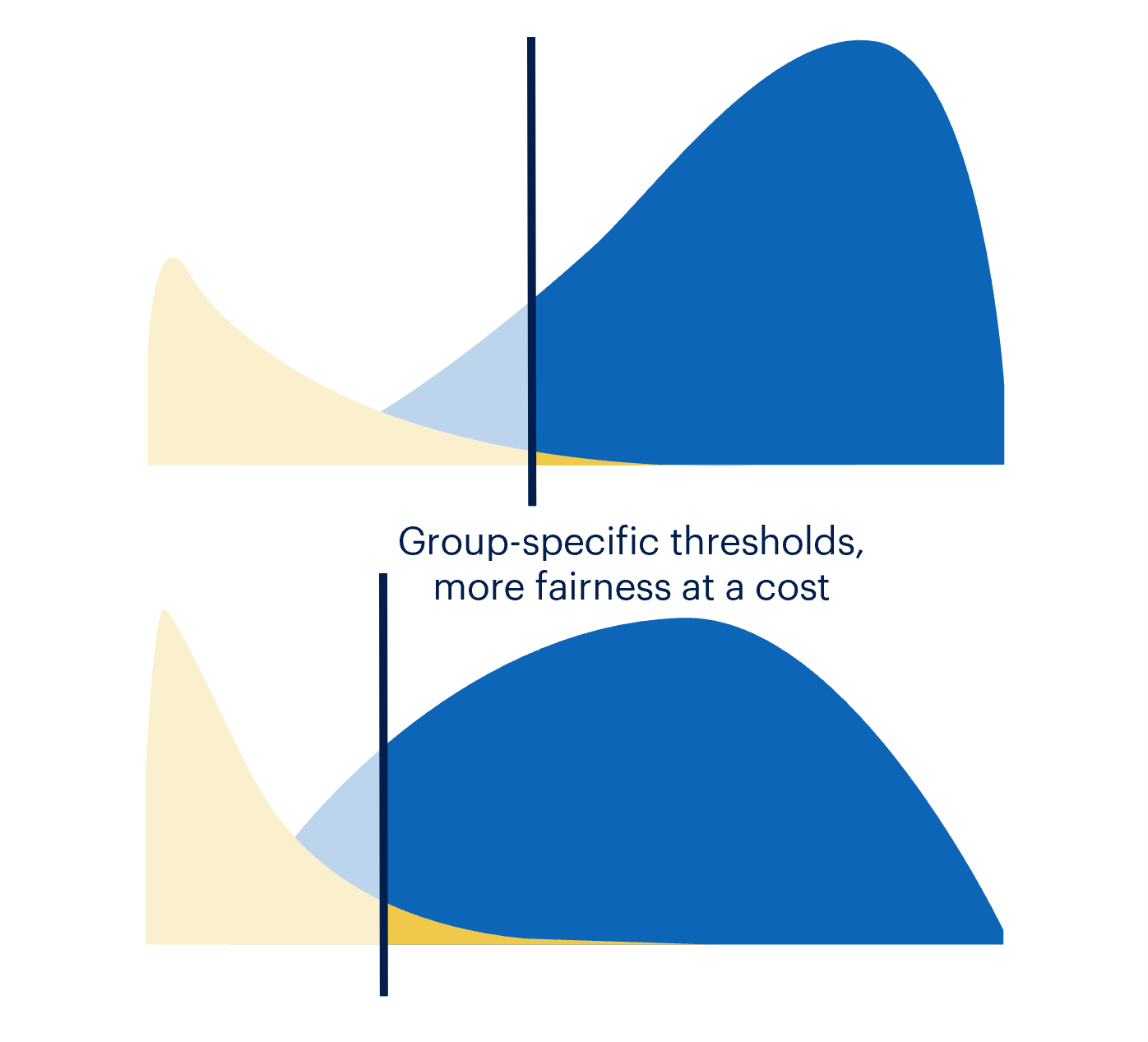
Putting It All Together: Model Improvement with Fairness Goals
How Do Outcomes Change for Regulators, Lenders, and Applicants?
We suggest combining the two approaches explored in our research: improving models using ML and setting fairness goals. While more advanced models don’t address disparities in credit access between groups, they do improve predictions generally. On the other hand, setting explicit fairness goals can address these disparities but not without a cost. Combining these two innovations softens the cost, which increases fairness and profitability relative to the current standard. This approach may balance some key concerns for regulators, lenders, and applicants.
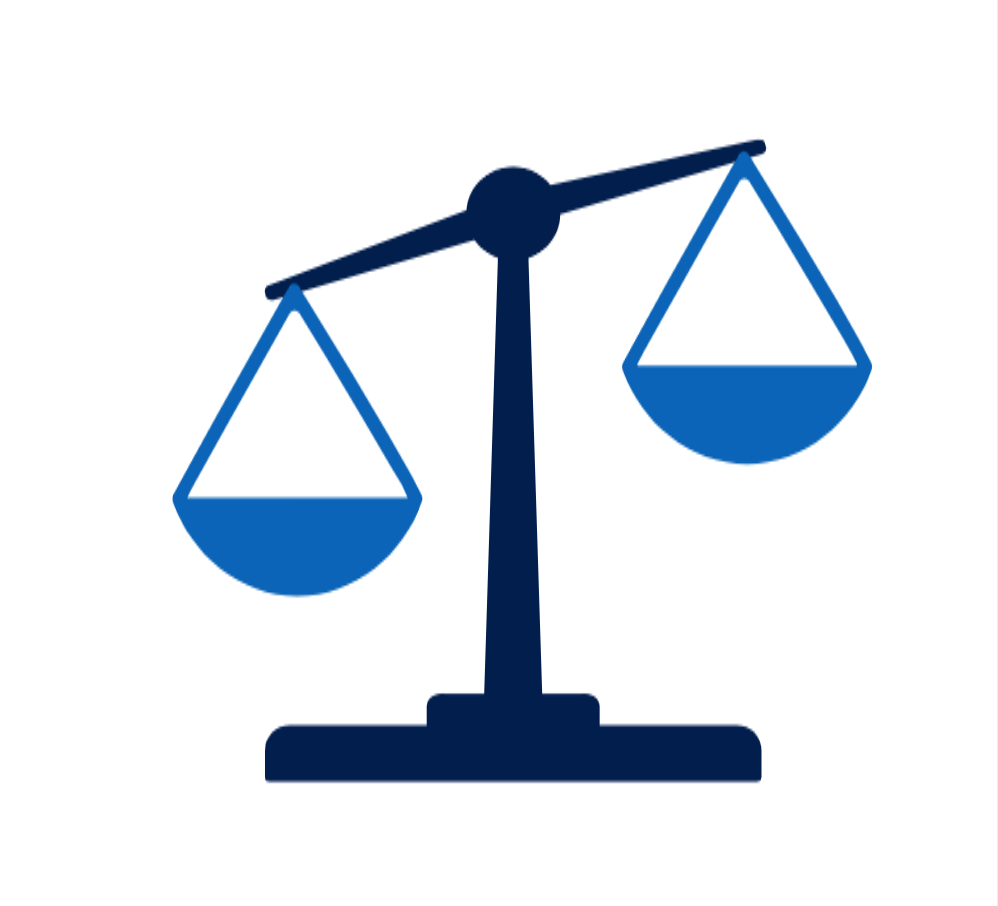
Documentation
About the Research
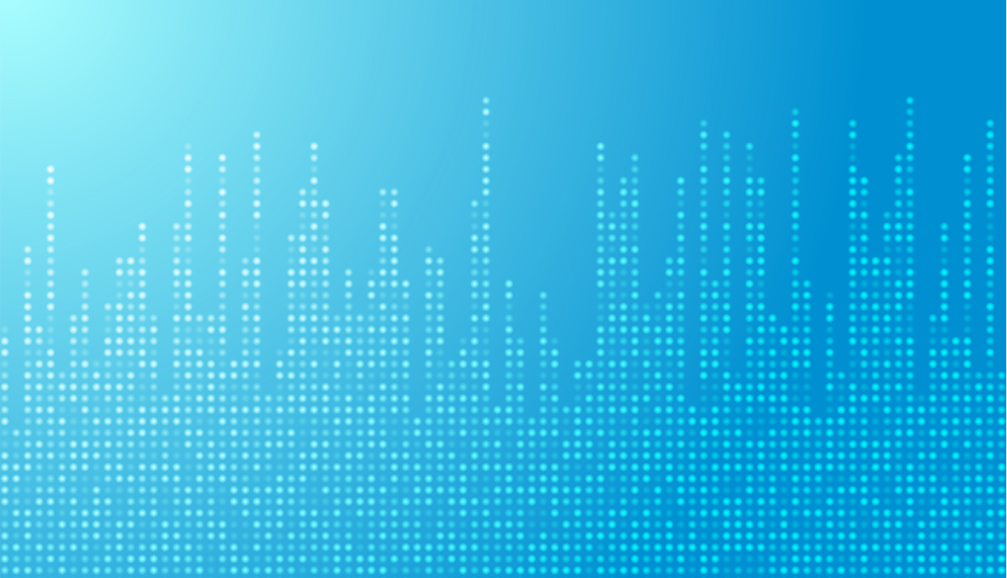
Contact the Team
- The views expressed here are solely those of the authors and do not necessarily reflect the views of the Federal Reserve Bank of Philadelphia or the Federal Reserve System.