Advancing Fairness in Lending Through Machine Learning
Putting It All Together: Model Improvement with Fairness Goals
Group-specific thresholds in combination with more advanced machine learning models can help reconcile regulator and lender interests; both fairness and profitability increase.
How Do Outcomes Change for Regulators, Lenders, and Applicants?
Advances in machine learning (ML) have paved the way for improved credit default predictions that can expand credit access to more applicants. However, more advanced ML models do not necessarily address disparities in credit access between groups. One solution to reduce the disparities discussed in our research is to set explicit fairness goals that are achieved through group-specific credit score thresholds. While group-specific thresholds can achieve fairer access to credit between groups, their use is associated with costs for lenders and borrowers. The last section examines how outcomes change when pairing the two approaches: improving models with ML and setting a fairness goal.
We highlight our findings through the lens of several key stakeholders: regulators, lenders, and applicants living in (LMI) and non-LMI areas. For simplicity and illustrative purposes, we assign specific considerations and related outcomes to each type of stakeholder; however, it’s important to recognize that the priorities of stakeholders overlap in reality. For example, the ongoing profitability of banks and other lenders is important to financial regulators, just as lenders value the fairness of their lending decisions.
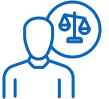
One consideration for regulators is fairness — whether there is equal access to credit among creditworthy applicants of different groups (e.g., LMI- and non-LMI-area applicants). When creditworthy applicants from both groups have equal access to credit, the (TPR) difference is near zero and the lending environment is fairer.
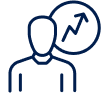
One consideration for lenders is making profitable lending decisions: balancing gains from repaid loans with the losses from loan defaults. To make a profit, lenders need to make multiple good loans for every defaulter because losses on defaults are much higher than profits from a good loan.1
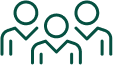
For applicants living in LMI areas, credit access is a major concern. Historically, LMI-area applicants have had lower access to credit compared with non-LMI-area applicants; therefore, an increase in their TPR, which translates to an increase in loans provided to creditworthy applicants, is favorable. Increases in the (FPR), however, are unfavorable since defaulting on a loan can be costly for a borrower, resulting, for example, in aggressive collections efforts, judicial action, and/or more difficulty accessing credit in the future. For applicants living in non-LMI areas, a policy that leaves applicants no worse off than existing policy is preferred (TPR no lower and FPR no higher).
An important highlight of this study is the fairness–profit tradeoff that focuses on considerations of regulators and lenders (see below). Our findings show that increasing fairness comes at a cost to profitability, but one that can be softened with the use of more advanced ML models. This tradeoff between fairness and profitability drives the need to find policy that can balance multiple priorities.
Try using the following interactive graphic to see how outcomes in our study change when altering model type and the fairness goal. Each selection is compared with the . The plot on the left illustrates the fairness–profit tradeoff, and the plots to the right showcase the specific outcomes of key stakeholders.
Overall, our research suggests that pairing advanced ML models and setting a fairness goal can be advantageous. Both fairness and profitability increase when using more advanced ML models and setting any fairness goal. Creditworthy applicants in LMI areas benefit from increased credit access, and non-LMI-area applicants are no worse off. An important cost to setting fairness goals alone is that more LMI-area applicants will default. This is costly to the LMI borrowers who default and to profitability for lenders. The use of advanced ML models helps to soften these costs but doesn’t eliminate them.
This research explores an alternative approach that uses ML and sensitive information to consciously reduce disparities between applicants who live inside and outside of LMI areas. The combination of embracing ML and setting explicit fairness goals may help address current disparities in credit lending and ensure that the gains from innovations in ML are more widely shared. To successfully achieve these goals, a broad conversation should continue with stakeholders such as lenders, regulators, researchers, policymakers, technologists, and consumers.
- We assume the lender requires revenue from four profitable loans (borrowers who repay) to make up for the loss from one charged-off loan (a borrower who defaults). This is a simplification intended to illustrate that a single defaulter is more costly than a single creditworthy applicant is profitable. Our results are robust to using other numbers.