We adopt the MA approach to forecast future observations and obtain the uniformly asymptotic expression for the mean squared prediction error (MSPE) of the averaging predictor. The MSPE can be decomposed into three components: non-stationary integration order, model complexity, and goodness-of-fit. The decomposition justifies that the advantage of MA comes from the diverse model intersections and provides the separation conditions under which the MA can attain strictly lower MSPE over model selection (MS). Regarding the predictive risk reduction by MA, it can be shown that the magnitude of MA improvement has the same order as the oracle minimum risk of MS under algebraic-decay case, while the magnitude is negligible under exponential-decay case. To pick the best choice of weights, we propose Shibata model averaging (SMA) criterion and show that, even without the integration order information, the selected weights by minimizing SMA and its variants including AIC-type and Mallow’s MA criteria are asymptotically optimal in the sense that: (i) The probability of a criteria minimizer with positive weights on models of dimension less than the integration order is negligible almost surely; (ii) The averaging predictor formed by the selected weights will ultimately achieve the lowest possible MSPE.
View the Full Working Paper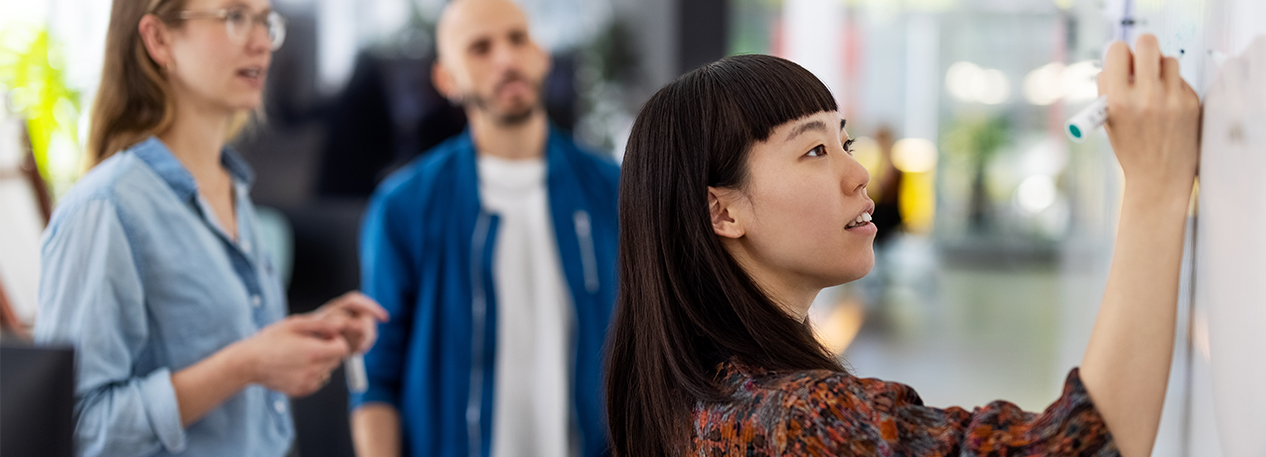
Working Paper
Model Averaging Prediction for Possibly Nonstationary Autoregressions
May 2023
WP 23-08 – This paper considers the problem of model averaging (MA) predictions for the integrated autoregressive processes of infinite order (AR(1)), which accommodates many stationary and nonstationary models in practice.