This revised brief fixes an error in the original brief published April 30, 2020, that led to incorrect numbers in Table 2. The error did not affect the numbers in other tables or substantively affect the findings and conclusions. These numbers have now been corrected.
The COVID-19 pandemic is affecting many people’s health and economic security. Official numbers show that over 26 million people filed for unemployment insurance (UI) during the first five weeks of the crisis.1 Recent studies have found that less educated, lower-income, and minority workers are at greatest risk of pandemic-related job loss, given the occupations and industries in which they work.2
This brief studies the economic impact of the pandemic through a different lens: that of place. We look in detail at the neighborhoods where residents are most at risk of job loss; at the initial economic, housing, and demographic characteristics of these residents; and at the impact of potential job loss on their economic and housing situation. We do so for the city of Philadelphia, the Philadelphia Federal Reserve District, and the nation as a whole.
Understanding these issues is important for many reasons. First, spatially concentrated impacts may be more disruptive than dispersed impacts and may place particular strain on local government and nonprofit resources. Second, even before the pandemic, neighborhoods differed dramatically in terms of incomes, housing costs, poverty rates, educational attainment, and other dimensions of inequality. If the crisis disproportionately impacts disadvantaged neighborhoods, it may worsen place-based inequalities. Finally, these answers can help identify potential gaps in existing policies and help direct needed resources to affected households during and after the crisis, promoting a more equitable recovery.
The rest of this brief explores these issues through three specific questions: Which neighborhoods are being affected? What are the characteristics of affected neighborhoods? What is the economic and housing situation of affected households?
Which Neighborhoods Are Being Affected?
To understand how neighborhoods are likely to be affected economically, we measure the share of all employment that is at risk because it requires close physical proximity and was therefore likely to be negatively impacted by social distancing requirements and associated shutdown orders.3 This measure is intended to capture all employment that is ultimately at risk because of the pandemic. By contrast, measures based on actual employment changes or UI claims better capture those already affected but are less able to capture those still at risk.4 We emphasize that the patterns described here are for employment by place of residence: These are the neighborhoods where people at risk of losing their jobs live, not where their jobs are located.
Figure 1. Employment At Risk for Neighborhoods in Philadelphia
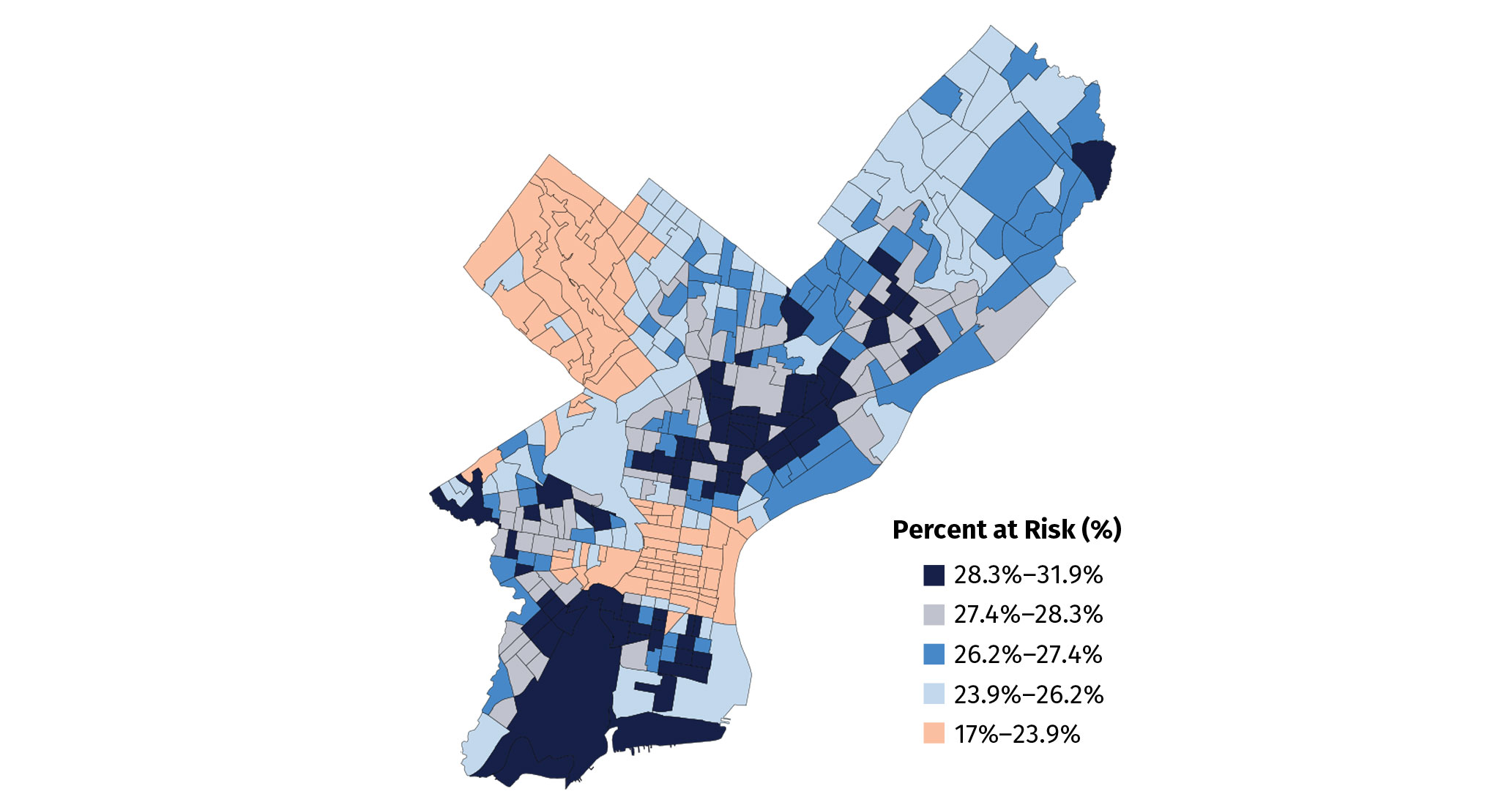
Figure 1 shows employment at risk by Philadelphia neighborhood (census tract). There are 384 neighborhoods in Philadelphia, and the map divides them into five equally sized groups based on the percent of employment that is at risk. The legend shows the risk values in each of these groups (quintiles). For example, risk in the first group ranges from 17 percent to 23.9 percent. Overall, the range is from a low of 17 percent to a high of 32 percent. However, the distribution is quite compressed in the middle three groups, ranging only from 24 to 28 percent, which is consistent with the pandemic having a broad impact.
Figure 1 does reveal differences in where the highest-risk (dark blue) and lowest-risk (light red) neighborhoods are located. The lowest-risk neighborhoods are concentrated in the central and northwest areas of the city, which are generally higher-income. The highest-risk neighborhoods are in the north central, northeast, and southwest neighborhoods, in generally lower-income and higher-poverty areas. We explore the characteristics of these and other neighborhoods in detail later.
Figure 2. Employment At Risk for Neighborhoods in the Philadelphia Federal Reserve District
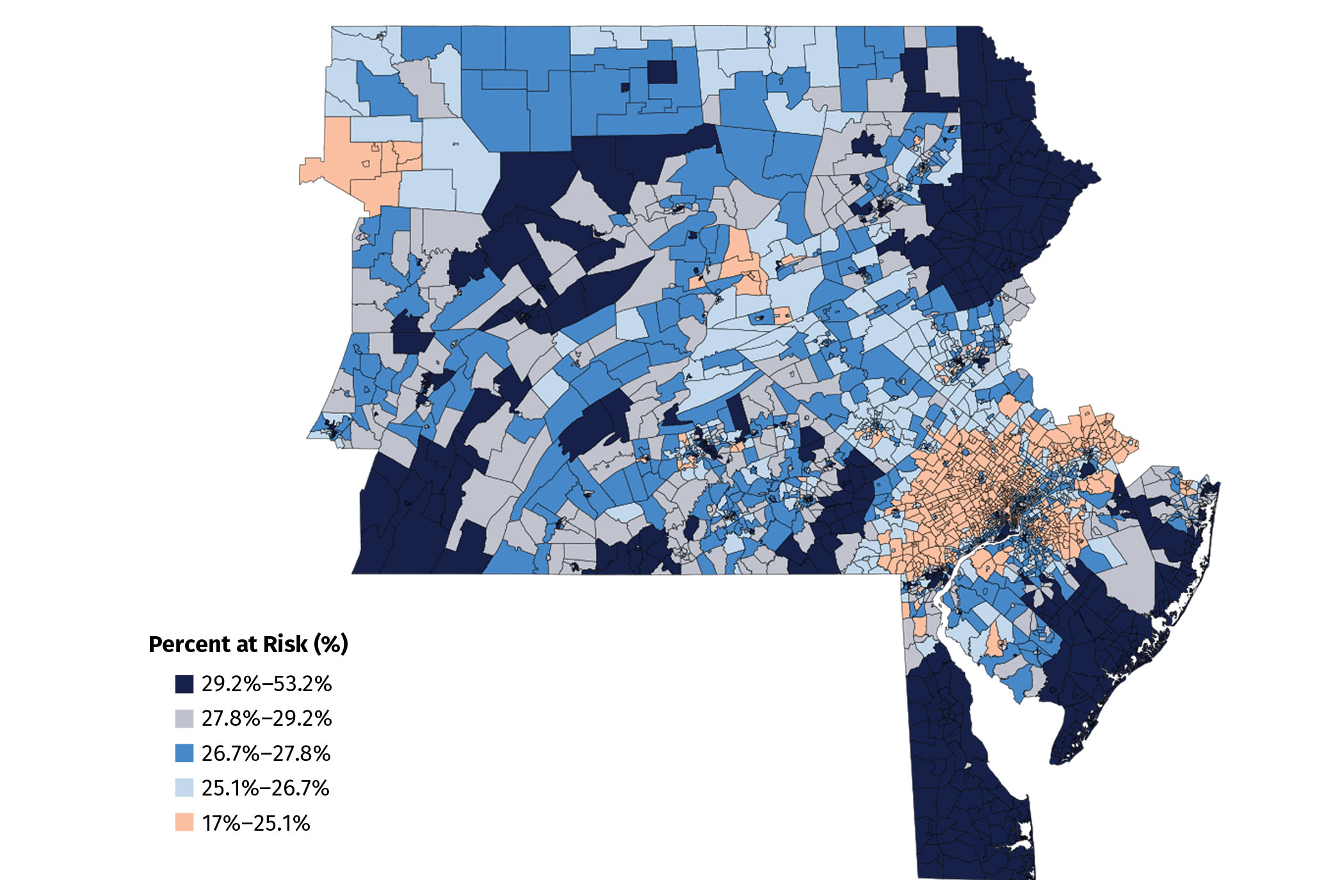
Figure 2 shows share at risk for the entire Philadelphia Federal Reserve District. The city of Philadelphia and surrounding suburbs are visible in the southeast. The suburbs west of Philadelphia are prominent among the lowest-risk neighborhoods, consistent with their being more educated and higher-income. By contrast, the highest-risk neighborhoods are located in southern Delaware, southern New Jersey, and northeastern Pennsylvania. Some rural parts of Pennsylvania also appear to be at highest risk. Although difficult to discern here, lower-income neighborhoods immediately east of Philadelphia (in Camden, NJ) and southwest of Philadelphia are also among those at highest risk.
Figure 3. Employment At Risk for Counties in the United States
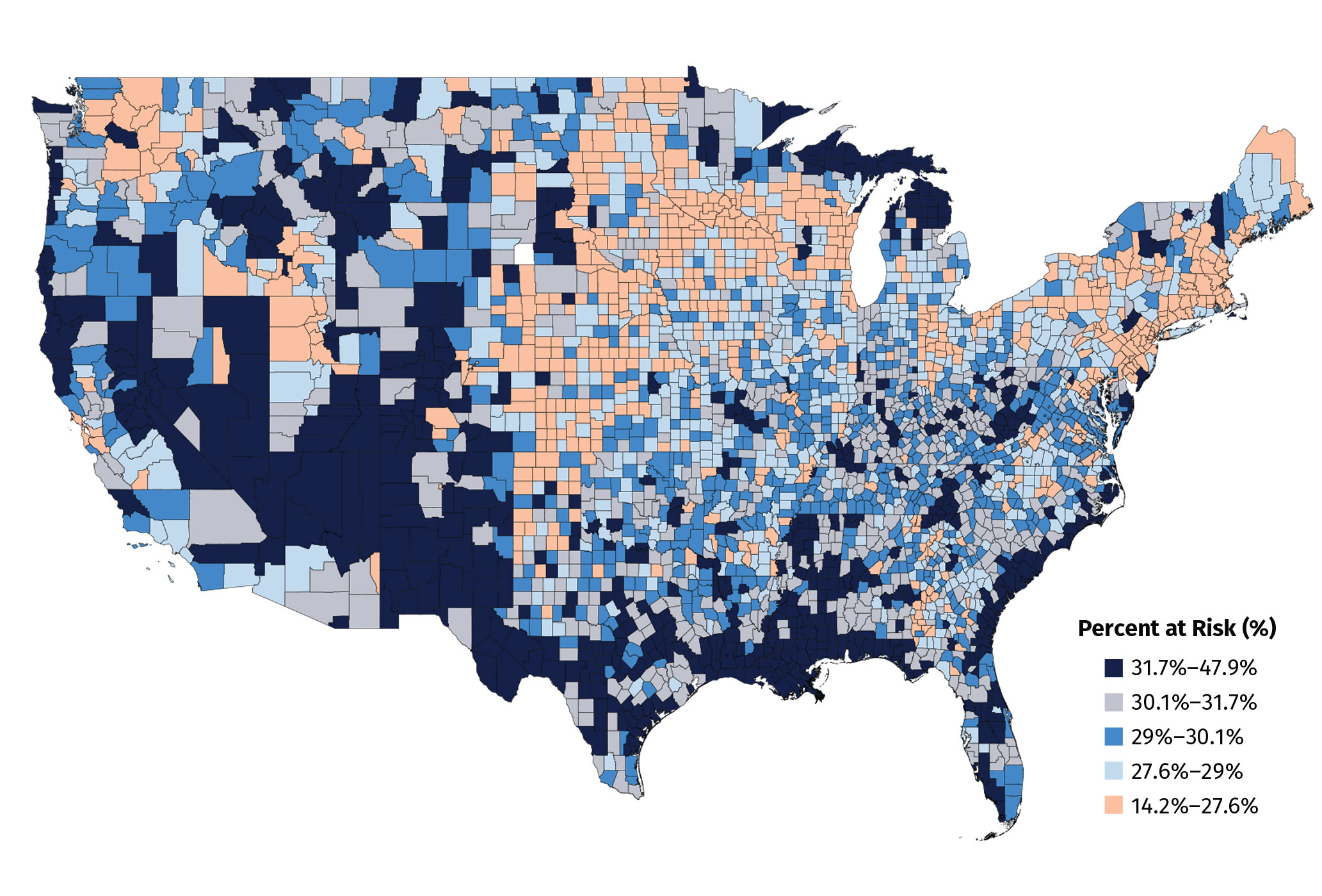
Figure 3 shows risk at the county level for all counties in the United States.5 The range of risk is similar to those in the Philadelphia Federal Reserve District, and the risk patterns are also somewhat similar: Coastal areas, particularly those outside large metropolitan areas in the Northeast, appear to be at highest risk, again consistent with tourism and hospitality being particularly impacted by the pandemic. By contrast, suburban counties of major metropolitan areas generally appear to be at lowest risk, consistent with them being more educated and higher-income. While some rural areas are at highest risk (in the Southwest), others are at lower risk (in the Midwest), making it difficult to draw broad conclusions about rural counties. Finally, it is worth emphasizing that state-level maps of these same data could look quite different, since very small and very populous dark blue areas (such as the counties of New York City) that are less prominent in this map could turn an entire state dark blue.6
Figures 1, 2, and 3 describe relative risk within each geography to facilitate comparisons within each map: for example, of high- and low-risk neighborhoods within Philadelphia. The tradeoff in this approach is that it makes it difficult to compare risk between maps: for example, how risk in Philadelphia neighborhoods might differ from risk in District or national neighborhoods. Figure 4 facilitates these comparisons by showing together the full risk distributions of neighborhoods in the city, District, and nation.
Figure 4. Distribution of Employment At Risk for Different Levels of Geography
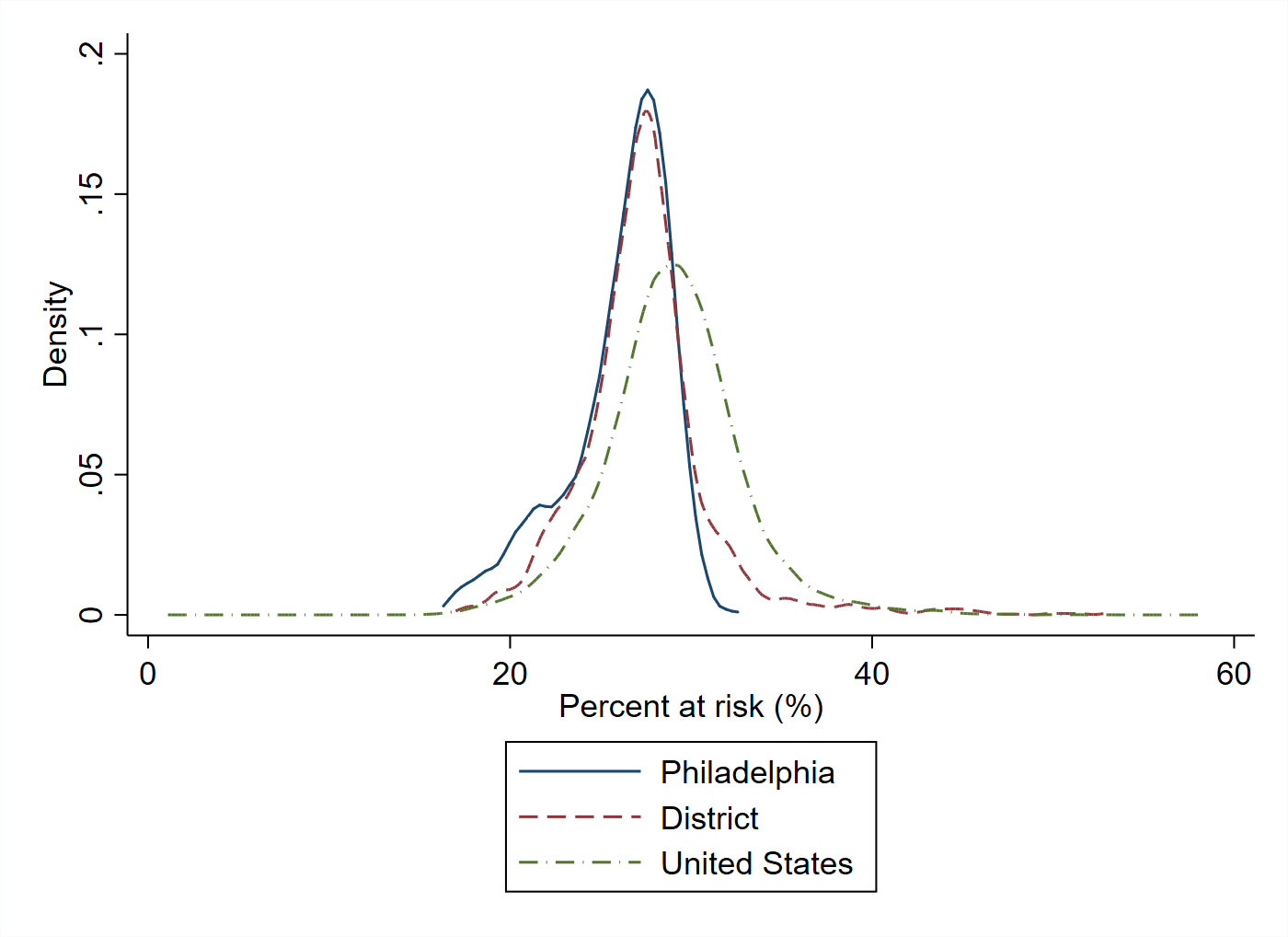
Figure 4 shows that the distribution of risk is reasonably similar in Philadelphia, the District, and nation, consistent with the broad impact of the pandemic. Risk does appear slightly higher in the nation as a whole, and the distribution is more dispersed, with long left and right tails. The long right tails in the District and nation suggest, as was evident in some of the maps, that certain parts of the District and nation include neighborhoods with employment concentrated in highly impacted industries (such as tourism and hospitality or energy). The long left tail nationally shows that at the same time, some national neighborhoods have workers who are highly concentrated in lower-risk industries, such as the technology sector.
What Are the Characteristics of Affected Neighborhoods?
In this section, we transition to exploring the demographic, socioeconomic, and housing characteristics of neighborhoods. We do so separately by neighborhood risk level and geography. This allows us to compare, for example, household incomes in the highest-risk neighborhoods in Philadelphia with those in the lowest-risk neighborhoods in Philadelphia and with those in the highest-risk neighborhoods in other parts of the country. Overall, these comparisons reveal that the highest-risk neighborhoods were more vulnerable to economic shocks even before the pandemic: Their residents had lower incomes, higher rent burdens, and were less likely to have health insurance. We now discuss these results in detail.
Table 1. Neighborhood Characteristics by Share of Employment At Risk
|
Philadelphia |
|
District |
|
United States | ||||||
---|---|---|---|---|---|---|---|---|---|---|---|
|
Highest Risk |
Middle Risk |
Lowest Risk |
|
Highest Risk |
Middle Risk |
Lowest Risk |
|
Highest Risk |
Middle Risk |
Lowest Risk |
At risk | 29.1 | 26.6 | 21.3 |
|
32.3 | 27.2 | 22.9 |
|
34.3 | 29 | 23.9 |
Bachelor’s degree (pct) | 13.3 | 22.2 | 67 |
|
23.8 | 24.2 | 55.3 |
|
20.3 | 25.8 | 52.8 |
Black (pct) | 42.4 | 50.1 | 17.8 |
|
11.8 | 15.6 | 9.3 |
|
18.5 | 13.7 | 7.8 |
Hispanic or Latino (pct) | 27.4 | 10.6 | 5.6 |
|
12.7 | 10.4 | 4.9 |
|
24.3 | 16.2 | 9.5 |
Asian (pct) | 8.3 | 5.6 | 9 |
|
2.7 | 2.8 | 7.5 |
|
3.2 | 4 | 9.4 |
Other (pct) | 2.1 | 2.7 | 3.8 |
|
2.4 | 2.1 | 2.3 |
|
4.5 | 3.3 | 3 |
White (pct) | 19.9 | 31 | 63.8 |
|
70.4 | 69.1 | 76.1 |
|
49.6 | 62.7 | 70.3 |
Poverty (pct) | 38.3 | 25.2 | 14.2 |
|
17.2 | 14.1 | 7.2 |
|
22.4 | 15.3 | 8.1 |
Income ($) | 31,372 | 43,423 | 77,451 |
|
56,653 | 60,779 | 101,282 |
|
46,716 | 60,178 | 99,852 |
Renters (pct) | 52.5 | 44.4 | 57 |
|
34.5 | 32.4 | 27.9 |
|
44.4 | 35.8 | 32.2 |
Median rent ($) | 911 | 989 | 1474 |
|
1043 | 1019 | 1453 |
|
960 | 1051 | 1516 |
Rent as pct. of income (pct) | 38.9 | 36 | 28.7 |
|
33.3 | 31.1 | 29.8 |
|
32.7 | 30.5 | 28.5 |
Rent as pct. of inc. >30% (pct) | 55.1 | 51.6 | 41.3 |
|
47.3 | 44.7 | 42.5 |
|
47.2 | 43.5 | 40 |
Rent as pct. of inc. >50% (pct) | 34.6 | 29.9 | 21.1 |
|
25.1 | 22.9 | 21.4 |
|
24.1 | 21.5 | 19.5 |
Median house value ($) | 108,849 | 155,718 | 416,861 |
|
210,266 | 177,914 | 371,222 |
|
185,216 | 222,495 | 463,503 |
With mortgage (pct) | 48.2 | 59 | 66.6 |
|
59.2 | 61.1 | 67.6 |
|
54.3 | 61.6 | 67.6 |
Owner cost of housing ($) | 673 | 929 | 1812 |
|
1141 | 1146 | 1871 |
|
904 | 1154 | 1881 |
Cost as pct. of income (pct) | 20.8 | 20.2 | 18.7 |
|
20.7 | 19.7 | 19.4 |
|
18.6 | 19 | 19.3 |
Cost as pct. of inc. >30% (pct) | 30.7 | 29.1 | 24.7 |
|
29 | 25.3 | 24.6 |
|
25.2 | 24.3 | 24.4 |
Cost as pct. of inc. >50% (pct) | 15.9 | 13.7 | 10 |
|
12.7 | 10.2 | 9.8 |
|
10.9 | 10.1 | 10.2 |
With health insurance (pct) | 87.9 | 91.1 | 94.9 |
|
91.7 | 92.9 | 96.3 |
|
85.8 | 90.7 | 95.3 |
Individuals in each nbhd. | 4,229 | 4,353 | 3,230 |
|
3,899 | 4,293 | 4,401 |
|
4,189 | 4,472 | 4,590 |
Households in each nbhd. | 1,448 | 1,594 | 1,515 |
|
1,450 | 1,640 | 1,669 |
|
1,491 | 1,653 | 1,779 |
Neighborhoods | 76 | 231 | 77 |
|
625 | 1,875 | 626 |
|
14,542 | 43,626 | 14,543 |
Table notes: Risk is the share of all employment at risk as defined previously. Highest risk: top quintile (20 percent) of neighborhood risk distribution within geographic. Middle risk: middle 3 quintiles (60 percent) of risk distribution. Lowest risk: bottom quintile (20 percent) of risk distribution. “Individuals” and “households in each neighborhood” are column averages. “Neighborhoods” is the count of all census tracts in each column. All figures are in 2019 dollars. Source: LEHD 2018 and American Community Survey 2014–2018 5-Year Estimates. Bachelor’s degree and the race/ethnicity variables are at the individual level. All others (poverty, income, rent variables, owner housing cost variables, and health insurance) are at the household level. |
Table 1 shows average neighborhood characteristics (in rows) for different levels of risk and geography (columns). Risk levels correspond to those in the maps described previous: highest-risk neighborhoods are those in the top quintile of risk (darkest blue), lowest-risk neighborhoods are those in the bottom quintile of risk (light red), and middle-risk neighborhoods are those in the middle three quintiles.7 To illustrate, the cell for row “Bachelor’s degree” in column “Philadelphia, Highest risk” has a value of 13.3. This means that among all 76 highest-risk neighborhoods in Philadelphia, the average share of adult residents having completed a bachelor’s degree or more is 13.3 percent. These 76 neighborhoods correspond to the darkest blue neighborhoods from Figure 1.
The first row shows the average of our at-risk measure. By construction, it is highest in the highest-risk category and lowest in the lowest-risk category. Consistent with the distribution in Figure 4, the average risk share in each risk category is slightly higher in the nation compared with Philadelphia, with the greatest difference in the highest-risk category (driven by the right tail of the risk distribution described in Figure 4). However, these distributions appear similar enough to allow meaningful comparisons of neighborhood characteristics between geographies.
The overall pattern in Table 1 is that neighborhoods that were more socioeconomically disadvantaged even before the pandemic are also at highest risk of employment loss because of the pandemic. This pattern is particularly striking in Philadelphia, consistent with greater initial levels of disadvantage and inequality within the city, but it is also evident in the District as a whole and in the nation. We discuss four dimensions of these patterns: socioeconomic status, race/ethnicity, housing, and health.
The highest-risk neighborhoods in Philadelphia have a bachelor’s degree completion rate of 13 percent, a poverty rate of 38 percent, and a median household income of $31,000. These numbers imply much greater initial disadvantage than in the lowest-risk Philadelphia neighborhoods (67 percent college completion, 14 percent poverty, $77,500 household income). They also show more disadvantage compared with the lowest-risk national neighborhoods (20 percent bachelor’s degree completion, 22 percent poverty, and $46,700 household income).
Similar patterns are present when looking at race/ethnicity. The highest-risk neighborhoods in Philadelphia are 42 percent black, 27 percent Hispanic or Latino, 8 percent Asian, and 20 percent white. By contrast, the lowest-risk neighborhoods in Philadelphia are 18 percent black, 6 percent Hispanic or Latino, 9 percent Asian, and 64 percent white. These findings are similar to those of other studies finding a greater economic impact on minority workers and show that there is also a greater impact on minority communities.
While the highest-risk neighborhoods in Philadelphia have the lowest monthly rent at $900, their lower incomes still result in the highest rent burdens: The average household spends 39 percent of income on rent, with 55 percent spending 30 percent or more (the standard definition of rent burden) and 35 percent spending at least half of their income on rent (the standard definition of severe rent burden). Rent burdens are much lower in the lowest-risk Philadelphia neighborhoods (consistent with those areas having much higher incomes despite also having higher rents) and also lower in the highest-risk District and national neighborhoods. Similar patterns for homeowners are less stark, although the share of homeowners who are severely cost burdened is higher in the highest-risk Philadelphia neighborhoods (16 percent) than in the lowest-risk Philadelphia neighborhoods (10 percent) or the highest-risk national neighborhoods (11 percent).
Finally, we observe similar patterns when looking at the share of neighborhood households with some form of public or private health insurance. Eighty-eight percent of households in the highest-risk Philadelphia neighborhoods have health insurance, while 95 percent of those in the lowest-risk Philadelphia neighborhoods have health insurance. These numbers are almost identical to those observed in the highest- and lowest-risk neighborhoods in the nation. This pattern is particularly worrisome, given those described previously: The households most at risk of job loss from the pandemic are also least likely to be insured against risk of illness from the pandemic, while also having fewer economic means to respond to such an illness.
What Is the Economic and Housing Situation of Affected Households?
Results from Table 1 show that neighborhoods with lower-income households, renters, and already cost-burdened households are at greatest risk of pandemic-related job loss. This section explores in greater detail the implications of job loss for the economic and housing situation of at-risk workers. The findings can help identify potential gaps in existing policy and how they can be addressed.
Table 2. Estimated Monthly Income, Expenses, and Deficits Before and After Job Loss for Households With At-Risk Workers
|
Number of At-Risk Households (000s) |
Before At-Risk Job Loss | After At-Risk Job Loss | ||||||
---|---|---|---|---|---|---|---|---|---|
Number with Deficit† (000s) |
Avg. Monthly Income Deficit* ($) |
Number with Deficit† (000s) |
Avg. Monthly Household Income* ($) |
Avg. Monthly Living Costs†* ($) |
Avg. Monthly Housing Costs* ($) |
Avg. Monthly Income Deficit†* ($) |
|||
Philadelphia | |||||||||
Renters | 57.9 | 20.4 | -1,029 | 43.1 | 572 | 1,172 | 1,070 | -1,669 | |
Homeowners (no mortgage) | 19.9 | 3.2 | -743 | 9.4 | 574 | 1,331 | 507 | -1,264 | |
Homeowners (with mortgage) | 41.9 | 7.1 | -886 | 21.9 | 1,047 | 1,455 | 1,379 | -1,787 | |
Total | 119.7 | 30.7 | -966 | 74.4 | 712 | 1,275 | 1,090 | -1,652 | |
District States | |||||||||
Renters | 701.7 | 220.4 | -1,049 | 504.2 | 698 | 1,345 | 1,154 | -1,802 | |
Homeowners (no mortgage) | 308.5 | 31.2 | -793 | 120.4 | 789 | 1,446 | 703 | -1,359 | |
Homeowners (with mortgage) | 856.4 | 95.9 | -1,253 | 367.0 | 1,390 | 1,684 | 1,897 | -2,191 | |
Total | 1,866.6 | 347.5 | -1,082 | 991.7 | 965 | 1,483 | 1,374 | -1,892 | |
United States | |||||||||
Renters | 11,487.0 | 3,946.9 | -1,038 | 8,551.3 | 711 | 1,430 | 1,100 | -1,819 | |
Homeowners (no mortgage) | 4,046.0 | 538.5 | -809 | 1,845.1 | 718 | 1,554 | 532 | -1,367 | |
Homeowners (with mortgage) | 10,733.1 | 1,377.7 | -1,167 | 4,976.7 | 1,310 | 1,746 | 1,659 | -2,094 | |
Total | 26,265.1 | 5,863.1 | -1,047 | 15,373.1 | 906 | 1,547 | 1,212 | -1,854 | |
* For households with deficit >$0. † Calculation uses adjusted living expenditures. See the Appendix for details. Table notes: All figures are in 2019 dollars. District State numbers are for all of Delaware, New Jersey, and Philadelphia because data limitations prevent estimates for the precise Philadelphia Federal Reserve District boundaries. Source: Authors’ calculations based on 2018 5YR ACS PUMS accessed through IPUMS, 2017 Consumer Expenditure Survey, 2019 Official USDA Food Plans, 2018 Medical Expenditures Panel Survey, and 2018 Kaiser Family Foundation State Health Facts. |
Table 2 combines individual income, household total income, household housing costs, and household living cost data for households with at least one worker identified as at risk of pandemic-related job loss.8 Individual and household Incomes and housing costs are self-reported from Census Bureau surveys. Household total income includes all most major public cash transfers and benefit programs — Temporary Assistance for Needy Families (TANF) and Supplemental Security Income (SSI) — with the exception of the Earned Income Tax Credit (EITC). Because self-reported living costs are not available in these surveys, we approximate these costs by adapting the methodology of the MIT Living Wage Calculator.9 We make additional adjustments for household composition, vehicle access, and self-reported public program participation (see the Appendix for details). These living costs include spending on food, health care, transportation, and other purchases (such as clothing) sufficient to meet a basic living standard.
Two important household resources not included in our calculations are savings and EITC amounts. While savings can certainly help households weather an economic shock, previous research shows that most low-income households, and many moderate-income households, have little in the way of savings.10 EITC amounts could be meaningful for our population of households with at-risk workers.11 The average annual EITC amount in 2017 for a family with children was $3,200, and many low-wage single parents may see amounts exceeding $5,000.12 Although the onset of the pandemic coincided with the regular tax filing season, difficulty accessing tax preparation services in light of widespread business and nonprofit closures may have limited households’ access to the EITC.
We first calculate the number of at-risk households with a “living income” deficit and their average deficit, even before a pandemic-related job loss. This deficit is calculated as household income from all sources minus self-reported housing costs minus the living cost estimates described previously. Column 2 shows that nationally, 5.9 million at-risk households experienced a deficit even before the pandemic, and Column 3 shows that the average monthly deficit among these households was with a deficit was $1,047.13 In Philadelphia, 30,700 at-risk households had an initial deficit. These results suggest that many at-risk households were unable to meet a “living wage” standard even before the pandemic. It is worth emphasizing that our calculations do not include all households, such as those without workers or those with workers in occupations not defined as at risk, who may nevertheless be struggling for reasons other than the risk of pandemic-related job loss.
We then calculate the number of at-risk households with a living income deficit after a pandemic-related job loss. To do this, we set every at-risk worker’s individual income to zero.14 We keep housing and health insurance costs constant and adjust all other living costs downward to reflect that households may be able to adjust along these margins in an economic emergency: by 15 percent for food, 80 percent for discretionary transportation costs,15 and 50 percent for all other spending.16
Column 4 shows that after job loss, the number of at-risk households with a living income deficit nationally increases to 15.4 million, which is an increase of 9.5 million (262 percent). Column 8 shows that their average deficit after job loss is $1,854. Among at-risk renter households in particular, the number with a deficit increases to 8.6 million, an increase of 4.6 million (117 percent). In Philadelphia, the number of at-risk households with a deficit after pandemic-related job loss is 74,400, an increase of 43,700 (143 percent). Their average deficit is approximately $1,652. Notably, the average household with a post–job loss deficit had a monthly income that was insufficient to cover even their housing costs. These estimates provide a sense of the scale of economic and housing insecurity created by the pandemic.
Although not shown in Table 2, we break out these numbers by race/ethnicity and household type and find inequalities along these dimensions as well. Nationally, at-risk householders of color were more likely to experience living income deficits both before and after pandemic-related job losses compared with white households. Before job loss, 32 percent of Hispanic or Latino householders, 31 percent of black householders, 27 percent of Asian householders, and 27 percent of Native American householders had income deficits, compared with 16 percent of white householders. After a job loss, 70 percent of Hispanic or Latino householders, 66 percent of black householders, 58 percent of Asian householders, and 63 percent of Native American householders would experience deficits, compared with 53 percent of white householders. Additionally, 53 percent of at-risk households headed by single women with children would have been unable to make ends meet prior to the crisis, with that share increasing to 78 percent after a job loss.
Finally, we consider how income provisions in the recent Coronavirus Aid, Relief, and Economic Security (CARES) Act may influence the scale of these numbers. The CARES Act expanded UI eligibility and generosity such that it now replaces up to 100% of lost income for up to four months for most workers, including contract, part-time, and self-employed workers. However, actual UI uptake is unlikely to reach 100% for various reasons even after these expansions, with one recent estimate suggesting it might only reach 64 percent nationally.17 Assuming that, of the 9.5 million new households we estimate will have living income deficits because of pandemic-related job loss, 64 percent receive 100 percent income replacement from UI and 36 percent do not, we can multiply 9.5 million by 36 percent to estimate that 3.4 million new households would have living income deficits, even taking UI into account. Similar calculations can be made using different numbers for the UI uptake rate. As our average monthly living income deficits before and after the pandemic show, other provisions of the CARES Act, such as one-time emergency payments, may help close remaining gaps, although the fact that they are one-time payments means that the timeline of social distancing requirements and economic recovery are important unknown factors.
Policy Implications
Our results have important implications for policy during and after the pandemic. First, we find that risk of pandemic-related job loss varies substantially by neighborhood and that the neighborhoods at highest risk were already among the most disadvantaged even before the pandemic. This implies that the pandemic has the potential to exacerbate preexisting place-based inequalities. Policymakers can help ensure that the aggregate policy responses at the national and state level are also appropriate for these communities, which were struggling the most before the pandemic and are now struggling the most because of it. Doing so will also promote a more equitable economic recovery after the pandemic.
Second, we help quantify the number of at-risk households living below a living wage standard of income both before the pandemic and after a pandemic-related job loss. Our finding that this number was 5.9 million nationally before the pandemic shows that many households with at-risk workers were already in a precarious position before the crisis. Our finding that it increases to 15.4 million after pandemic-related job losses, an increase of 9.5 million households, shows just how much the pandemic may be contributing to economic and housing insecurity. Similar to our neighborhood-level analyses, we find that certain households, such as minority households, have been particularly impacted. Our methodology and results can help inform policymakers and advocates seeking to improve economic and housing security before, during, and after the pandemic.
Federal legislation and a patchwork of state and local efforts have extended temporary relief from rent and mortgage obligations to many households. However, it is still unknown how these households will pay rental and mortgage arrears once these temporary measures are lifted. Our results suggest that these accumulated arrears could be substantial. While national expansions of UI generosity will go a long way toward replacing income for the households receiving UI, the likelihood that UI uptake will not reach 100 percent suggests that additional income replacement, housing cost subsidies, or other policies may be required to keep affected households in their homes.
These results point to important questions for policymakers, researchers, and advocates to address. Differences in the impact of the pandemic by place suggest that while federal policies such as the CARES Act have been crucial to mitigating the economic impact of the pandemic, they may not be sufficient for every region, city, or neighborhood. Local governments and nonprofits are well placed to identify potential gaps in existing policies and implement local solutions, for example, as they have done in areas such as rent payment and evictions. However, the economic fallout from the pandemic has created tremendous strain in local budgets, potentially impairing the ability of local governments to fulfill this important role. This reinforces the importance of investing in the capacity of local governments and nonprofits to close policy gaps and ensure an equitable recovery.
- U.S. Department of Labor Unemployment Insurance Weekly Claims reports.
- For example, Keith Wardrip and Anna Tranfaglia (2020), Which Workers Will Be Most Impacted?
- We use publicly available 2018 employment data from the Census Bureau LEHD. It includes counts of employment by neighborhood (census tract) of residence and industry. We create industry-level risk using the occupation-level risk measure from a previous brief in this series (Wardrip and Tranfaglia, 2020). It classifies occupations requiring close physical contact as at-risk, excluding workers in health care and education, grocery stores and pharmacies, and local, state, and federal government.
- An additional benefit of our measure, which is crucial to this brief, is our ability to construct it at the neighborhood level. By contrast, employment and unemployment data are typically only available at the state or county level.
- We exclude Alaska and Hawaii for display purposes.
- While a state-level map would be informative for different reasons (since states are important administrative units), we prefer this county-level map to better highlight heterogeneity in risk within states.
- Also as in the maps, the national sample includes the neighborhoods in the Philadelphia Federal Reserve District and city of Philadelphia samples, and the District sample includes the neighborhoods in the city sample.
- The worker-level at-risk measure is from Wardrip and Tranfaglia (2020) and identifies workers in occupations that require physical proximity, with certain exclusions for essential workers. It is the same measure used to construct our neighborhood-level risk measure. The risk measure and other data sources are described in detail in the Appendix.
- “The living wage model is an alternative measure of basic needs. It is a market-based approach that draws upon geographically specific expenditure data related to a family’s likely minimum food, childcare, health insurance, housing, transportation, and other basic necessities (e.g., clothing, personal care items, etc.) costs,” livingwage.mit.edu/pages/about.
- Report on the Economic Well-Being of U.S. Households in 2018, www.federalreserve.gov/publications/2019-economic-well-being-of-us-households-in-2018-dealing-with-unexpected-expenses.htm.
- Pretax transfers, such as TANF, SNAP, SSI, and Social Security, are included in our household income category.
- Center on Budget and Policy Priorities, http://www.cbpp.org/research/federal-tax/policy-basics-the-earned-income-tax-creditwww.cbpp.org/research/federal-tax/policy-basics-the-earned-income-tax-credit.
- These deficits are consistent with results from other studies using other sources of income and living costs that show many low-income Americans have costs that exceed expenses and little to no savings. For example, the Consumer Expenditures Survey 2018 shows that many people’s actual expenses exceed income after taxes, particularly for lower-income people. While our median deficits appear high, it is worth emphasizing a point made previously: this is income relative to a “living wage” cost standard. Our results show that many at-risk households were not reaching that standard before the pandemic, perhaps because they opted to forgo spending on key goods and services, such as adequate food and health insurance. Other parts of the gap could be explained by borrowing, by drawing down any existing savings, or by EITC and other unrecorded cash transfers or in-kind benefits.
- If there are workers in the household not identified as at risk according to our measure, we leave their income as it is.
- For households with vehicles, this 80 percent reduction is applied to gas and motor oil expenses. For households without vehicles, this 80 percent reduction is applied to public transit and other transportation expenses.
- Details are in the Appendix. Although not shown here, our results are very similar when we adjust living costs after job loss by more or less, including not at all. This suggests that this assumption is not driving our key results.
- Parrott and Stettner (2020). Their uptake estimate is based on historical state-level uptake rates, which are then adjusted upward to account for expanded eligibility and the unique nature of the pandemic. The final national adjusted estimate is 64 percent, with state-level adjusted estimates ranging from 51 percent to 81 percent: tcf.org/content/commentary/covid-stimulus-3-0-ui-reaction/.