Because set identification can be challenging, they propose to include micro data on heterogeneous entities to sharpen inference. First, the authors provide conditions when imposing a simple ranking of impulse-responses sharpens inference in bivariate and trivariate VARs. Importantly, they show that this set reduction also applies to variables not subject to ranking restrictions. Second, the authors develop two types of inference to address recent criticism: (1) an efficient fully Bayesian algorithm based on an agnostic prior that directly samples from the admissible set and (2) a prior-robust Bayesian algorithm to sample the posterior bounds of the identified set. Third, they apply our methodology to U.S. data to identify productivity news and defense spending shocks. The authors find that under both algorithms, the bounds of the identified sets shrink substantially under heterogeneity restrictions relative to standard sign restrictions.
View the Full Working Paper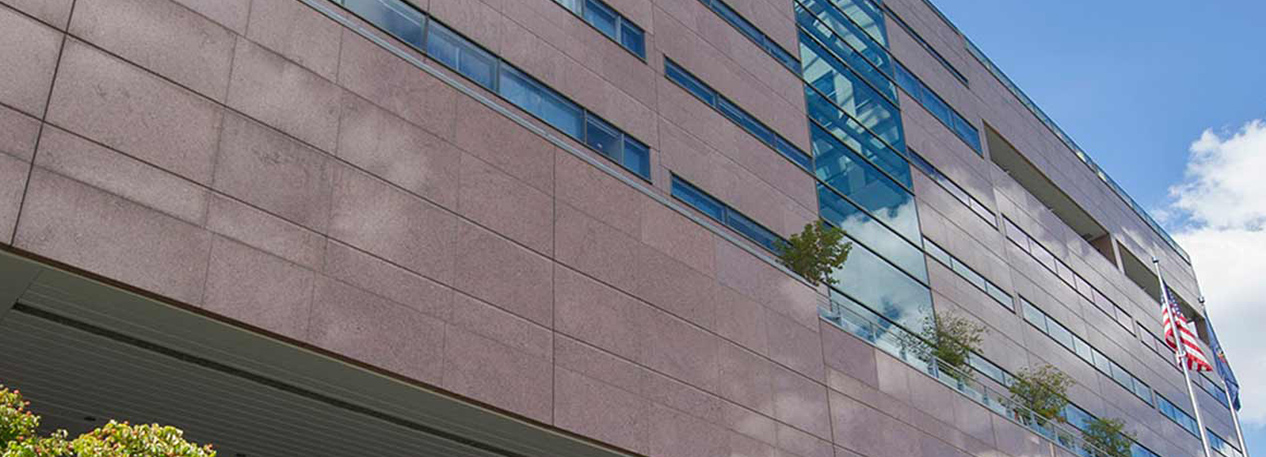
Working Paper
Identification Through Heterogeneity
April 2017
WP 17-11 - The authors analyze set identification in Bayesian vector autoregressions (VARs).