The key issue is this: In the literature on developing forecasting models, new models are put together based on the results they yield using the data set available to the model’s developer. But those are not the data that were available to a forecaster in real time. How much difference does the vintage of the data make for such forecasts? The authors explore this issue with a variety of exercises designed to answer this question. In particular, they find that the use of real-time data matters for some forecasting issues but not for others. It matters for choosing lag length in a univariate context. Preliminary evidence suggests that the span — or number — of forecast observations used to evaluate models may also be critical: the authors find that standard measures of forecast accuracy can be vintage-sensitive when constructed on the short spans (five years of quarterly data) of data sometimes used by researchers for forecast evaluation. The differences between using real-time and latest-available data may depend on what is being used as the “actual” or realization, and the authors explore several alternatives that can be used. Perhaps of most importance, the authors show that measures of forecast error, such as root-mean-squared error and mean absolute error, can be deceptively lower when using latest-available data rather than real-time data. Thus, for purposes such as modeling expectations or evaluating forecast errors of survey data, the use of latest-available data is questionable; comparisons between the forecasts generated from new models and benchmark forecasts, generated in real time, should be based on real-time data.
View the Full Working Paper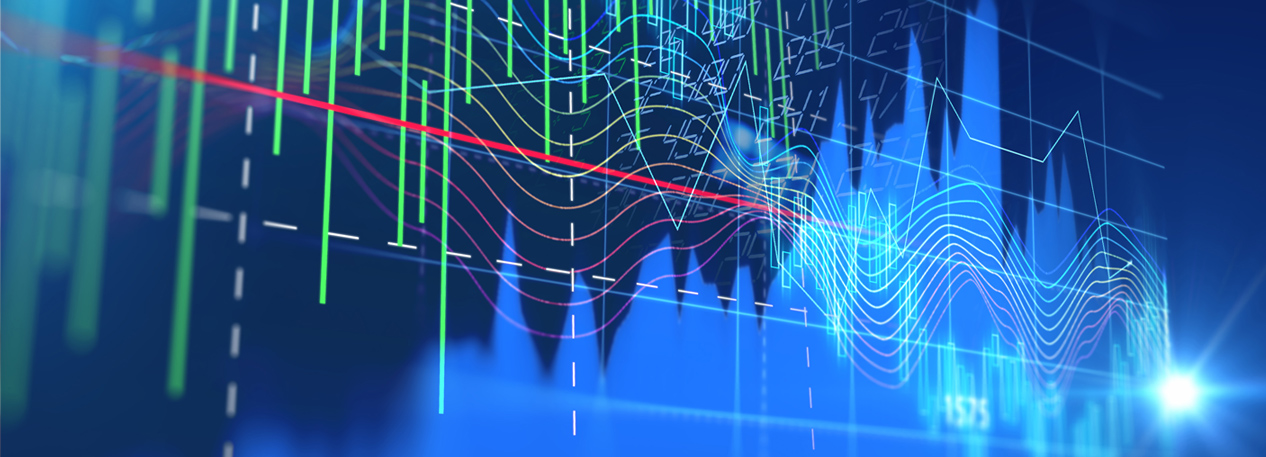
Working Paper
Forecasting with a Real-Time Data Set for Macroeconomists
July 2001
WP 01-10 – This paper discusses how forecasts are affected by the use of real-time data rather than latest-available data.
Share
Download